
The goal of this tutorial is to familiarize readers with the logic of SSEDs and how they can be used to establish evidence-based practice. The basics of SSED methodology are described, followed by descriptions of several commonly implemented SSEDs, including their benefits and limitations, and a discussion of SSED analysis and evaluation issues. Finally, a number of current issues in SSEDs, including effect size calculations and the use of statistical techniques in the analysis of SSED data, are considered. The first author (referred to as the interventionist) conducted and collected data for the study.
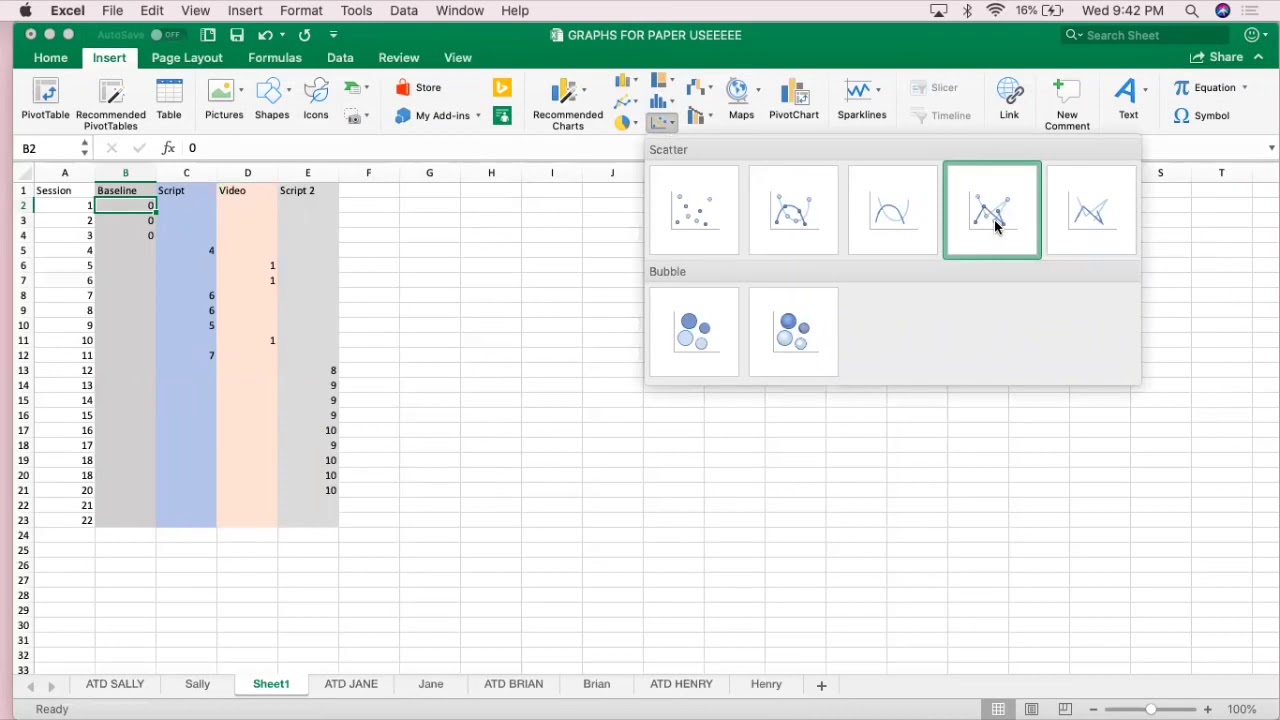
Additional information
In addition, the carefully controlled conditions in which RCTs must be conducted to ensure that the results are interpretable may not be comparable and/or possible to implement in real-life (i.e., uncontrolled) conditions. SSEDs are an ideal tool for establishing the viability of treatments in real-life settings before attempts are made to implement them at the large scale needed for RCTs (i.e., scaling up). Ideally, several studies using a variety of methodologies will be conducted to establish an intervention as evidence-based practice. When a treatment is established as evidence based using RCTs, it is often interpreted as meaning that the intervention is effective with most or all individuals who participated. Thus, systematic evaluation of the effects of a treatment at an individual level may be needed, especially within the context of educational or clinical practice. SSEDs can be helpful in identifying the optimal treatment for a specific client and in describing individual-level effects.
Withdrawal (ABA and ABAB) Designs
For example, if one's objective were to teach or establish a new behavior that an individual could not previously perform, returning to baseline conditions would not likely cause the individual to “unlearn” the behavior. Similarly, studies aiming to improve proficiency in a skill through practice may not experience returns to baseline levels when the intervention is withdrawn. In other cases, the behavior of the parents, teachers, or staff implementing the intervention may not revert to baseline levels with adequate fidelity. In other cases still, the behavior may come to be maintained by other contingencies not under the control of the experimenter.
Interventionist
The last five sessions were conducted beyond the aforementioned 50 % sessions rule (see the “Prompt Hierarchy Comparison and Target Reassignment” section) because an ascending trend in correct responding was observed. During the additional sessions, responding returned to a lower level and the intervention was then discontinued. The control condition targets were also reassigned to MTL prompting, and James mastered these targets with MTL prompting in 13 sessions.
The authors concluded that the MTL procedure with a time delay should be the default procedure used for teaching skills to children with ASD whose instructional history is unknown. Multiple-baseline and multiple-probe designs are appropriate for answering research questions regarding the effects of a single intervention or independent variable across three or more individuals, behaviors, stimuli, or settings. On the surface, multiple-baseline designs appear to be a series of AB designs stacked on top of one another. However, by introducing the intervention phases in a staggered fashion, the effects can be replicated in a way that demonstrates experimental control. In a multiple-baseline study, the researcher selects multiple (typically three to four) conditions in which the intervention can be implemented. The intervention is introduced systematically in one condition while baseline data collection continues in the others.
If the multiple baselines are being conducted across behaviors, those behaviors must be similar in function, topography, and the effort required to produce them while remaining independent of one another. Withdrawal designs (e.g., ABA and ABAB) provide a high degree of experimental control while being relatively straightforward to plan and implement. However, a major assumption of ABAB designs is that the dependent variable being targeted is reversible (e.g., will return to pre-intervention levels when the intervention is withdrawn). If the individual continues to perform the behavior at the same level even though the intervention is withdrawn, a functional relationship between the independent and dependent variables cannot be demonstrated. When this happens, the study becomes susceptible to the same threats to internal validity that are inherent in the AB design. By replicating an investigation across different participants, or different types of participants, researchers and clinicians can examine the generality of the treatment effects and thus potentially enhance external validity.
The authors discuss the requirements of each design, followed by advantages and disadvantages. The logic and methods for evaluating effects in SSED are reviewed as well as contemporary issues regarding data analysis with SSED data sets. Specific exemplars of how SSEDs have been used in speech-language pathology research are provided throughout. Single-subject experimental designs (SSEDs) represent an important tool in the development and implementation of evidence-based practice in communication sciences and disorders.
Multiple-Treatment Designs
If this is the first time you used this feature, you will be asked to authorise Cambridge Core to connect with your Dropbox account.Find out more about saving content to Dropbox.
Abstract
Design of an alternating distributed large and small amounts of CNT/polyether amine coating on carbon fiber to derive ... - ScienceDirect.com
Design of an alternating distributed large and small amounts of CNT/polyether amine coating on carbon fiber to derive ....
Posted: Wed, 08 Feb 2023 08:00:00 GMT [source]
For instance, the conservative dual criterion fits a mean line and a trend line to the baseline data and extends them into the intervention phase for comparison (Fisher et al., 2003). The conservative dual criterion can be considered a visual aid, as suggested by its authors, but it actually entails obtaining a p-value (i.e., the probability of observing, only by chance, as many or more intervention points superior to both extended baseline lines, as the number actually observed). The p-value is not a quantification of the reliability or the replicability of the results (Branch, 2014). In fact, p-values do not preclude replications or make them unnecessary, because they are not a tool for extrapolating the results to other participants. Comparing data paths is common in visual analysis of graphed SCED data, and in many ways relies on implicit use of interpolated values between sessions for each data path.
As a result, any increases observed during the intervention phase may simply be a continuation of that trend rather than the result of the manipulation of the independent variable. This underscores the importance of “good” baseline data, and, in particular, of the need to continue collecting baseline data to eliminate the possibility that any trends observed are likely to continue in the absence of an intervention. The use of single-subject experimental designs (SSEDs) has a rich history in communication sciences and disorders (CSD) research. A number of important studies dating back to the 1960s and 1970s investigated fluency treatments using SSED approaches (e.g., Hanson, 1978; Haroldson, Martin, & Starr, 1968; Martin & Siegel, 1966; Reed & Godden, 1977). Several reviews, tutorials, and textbooks describing and promoting the use of SSEDs in CSD were published subsequently in the 1980s and 1990s (e.g., Connell, & Thompson, 1986; Fukkink, 1996; Kearns, 1986; McReynolds & Kearns, 1983; McReynolds & Thompson, 1986; Robey, Schultz, Crawford, & Sinner, 1999). Despite their history of use within CSD, SSEDs are sometimes overlooked in contemporary discussions of evidence-based practice.
The inclusion of randomization of condition ordering in the design also allows the investigator to use a specific analytical technique called randomization tests (Edgington, 1967, 1975). Randomization tests are applicable across different kinds of SCEDs (Craig & Fisher, 2019; Heyvaert & Onghena, 2014; Kratochwill & Levin, 2010), as long as there is randomization in the design, such as the random assignment of conditions to measurement occasions (Edgington, 1980; Levin et al., 2019). Randomization tests are also flexible in the selection of a test statistic according to the type of effect expected (Heyvaert & Onghena, 2014). In particular, the test statistic can be defined according to whether the effect is expected to be a change in level or in slope (Levin et al., 2020), and whether the change is expected to be immediate or delayed (Levin et al., 2017; Michiels & Onghena, 2019). The test statistic is just the computation of a specific measure of the difference between conditions that is of interest to the researcher for which a p-value will be obtained. Owing to the presence of randomization in condition ordering, there is no need to refer to any theoretical sampling distribution that would require random sampling.
All participants were 5 years old and were enrolled full time in a preschool for children with ASD that based instruction on the principles of behavior analysis. The school’s clinical director and teachers referred participants to the interventionist because the students exhibited difficulties acquiring skills. In particular, the staff reported that the participants demonstrated difficulty learning one-step directions. In 2 years prior to the study, James acquired only two one-step directions, Joseph did not acquire any one-step directions, and Sean acquired three one-step directions. In addition, teaching participants to follow one-step directions may be conceived as a behavioral cusp (Rosales-Ruiz & Baer 1997), functioning as a prerequisite skill for more advanced behaviors. Research has demonstrated that most-to-least (MTL) and least-to-most (LTM) prompting are effective in helping children with Autism Spectrum Disorders acquire a variety of new skills.
Although, in theory, these types of designs can be extended to compare any number of interventions or conditions, doing so beyond two becomes excessively cumbersome; therefore, the alternating treatments design should be considered. Because replication of the experimental effect is across conditions in multiple-baseline/multiple-probe designs, they do not require the withdrawal of the intervention. This can make them more practical with behaviors for which a return to baseline levels cannot occur. Depending on the speed of the changes in the previous conditions, however, one or more conditions may remain in the baseline phase for a relatively long time.
This design involves systematically and alternatingly implementing multiple treatments, allowing for quick evaluation and comparison of the outcomes of each treatment. Note also that lost in the above discussion concerning effect size metrics is the issue of statistical versus clinical significance. From a practice perspective, one of the problems of statistical significance is that it can over- or underestimate clinical significance (Chassan, 1979). One argument for statistically analyzing single-subject data sets, mentioned above, is that visual inspection is prone to Type 1 error in the presence of medium to small effects (Franklin et al., 1996). Unfortunately, the proposed solution of implementing conventional inferential statistical tests with single-subject data based on repeated measurement of the same subject is equally prone to Type 1 error because of autocorrelation.
No comments:
Post a Comment